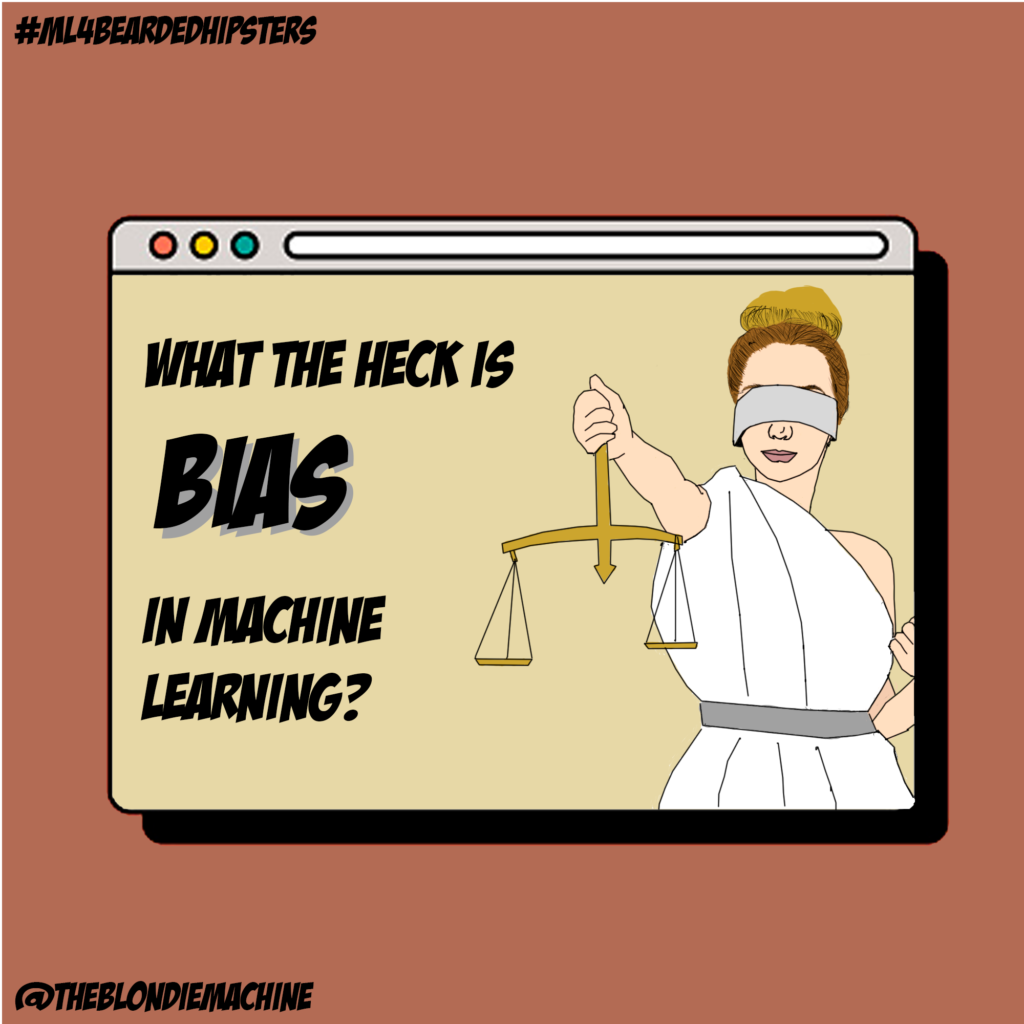
This week is the turn of a new instructional comic of the Machine Learning 4 Bearded Hipsters series (#ML4BeardedHipsters). This time we will talk about BIAS, a thing that may seem inoffensive but can transform your models in imprecise models and also racist and sexist models.
But, what the heck is bias?
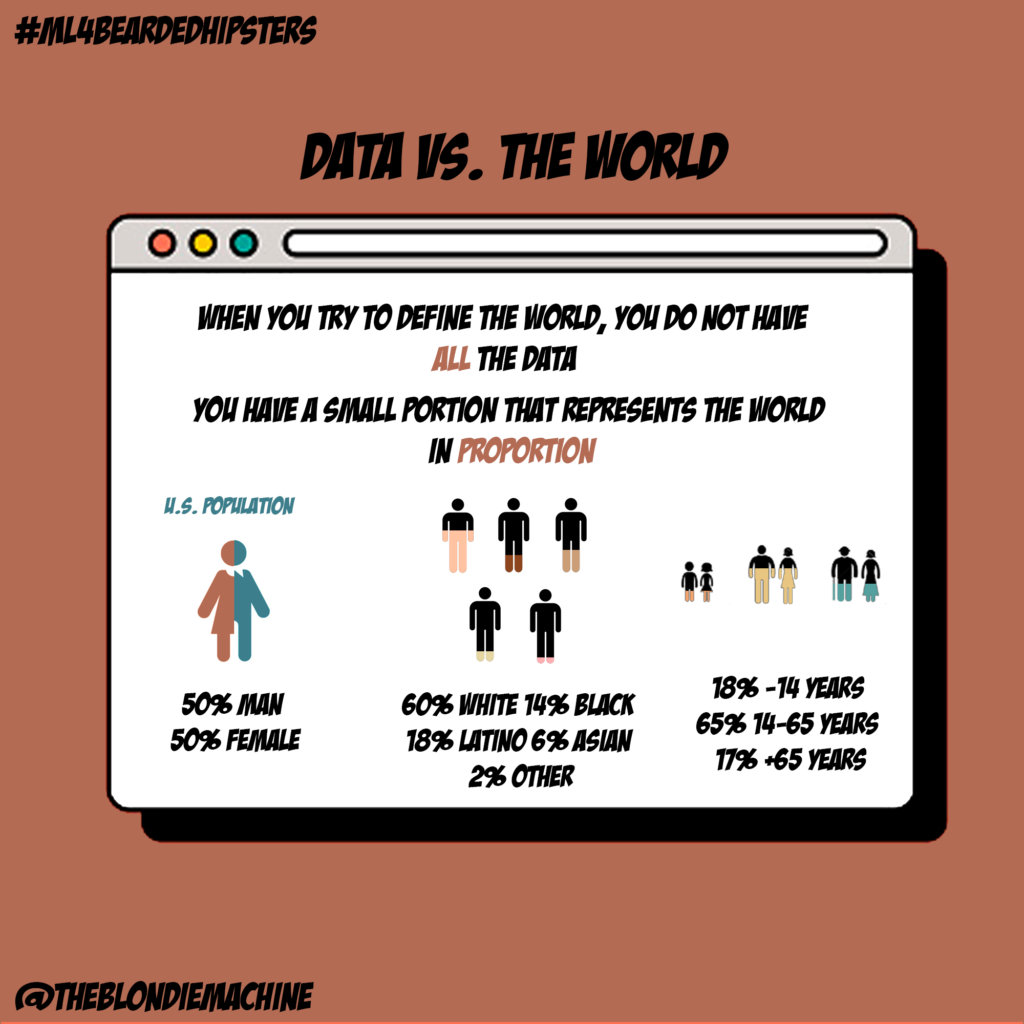
When you try to train a model from data, you model it according to the training data. But, is your training data representative and well balanced with the real-world situations you are trying to model?
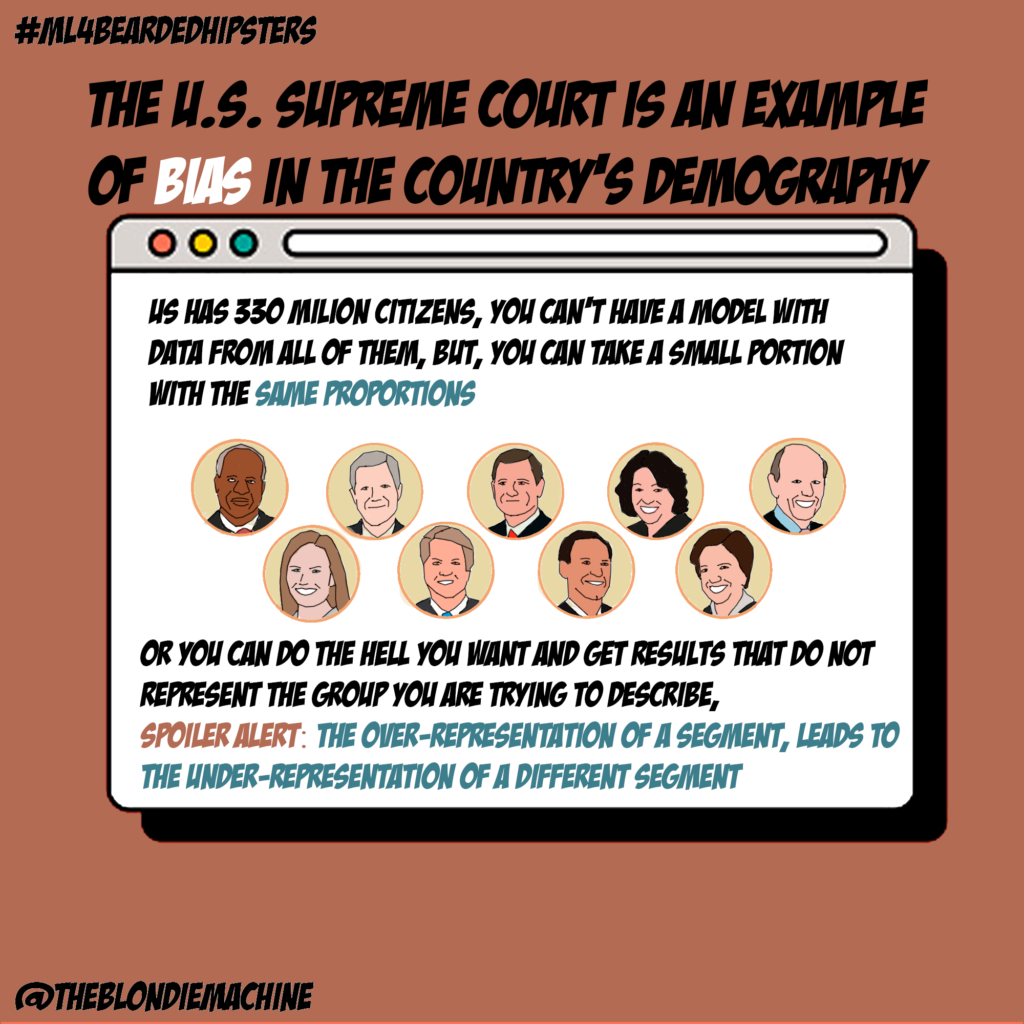
If you want your model to work well with real-world data, and you do not have access to ALL the data, its ok. But when you recollect your data, be sure it its well proportioned with the total of what you are trying to describe. Because, if you miss represent a segment of your data it will automatically over represent at least one of the remaining sectors. In game and economics theory this is called a zero-sum game. If one segment gains representation, another segments need to lose it.
Types of bias
There are different types, some can be accidental and some intentional, but all of them are equally dangerous to your data. Since models tend to accentuate the bias in the final representation of the data.
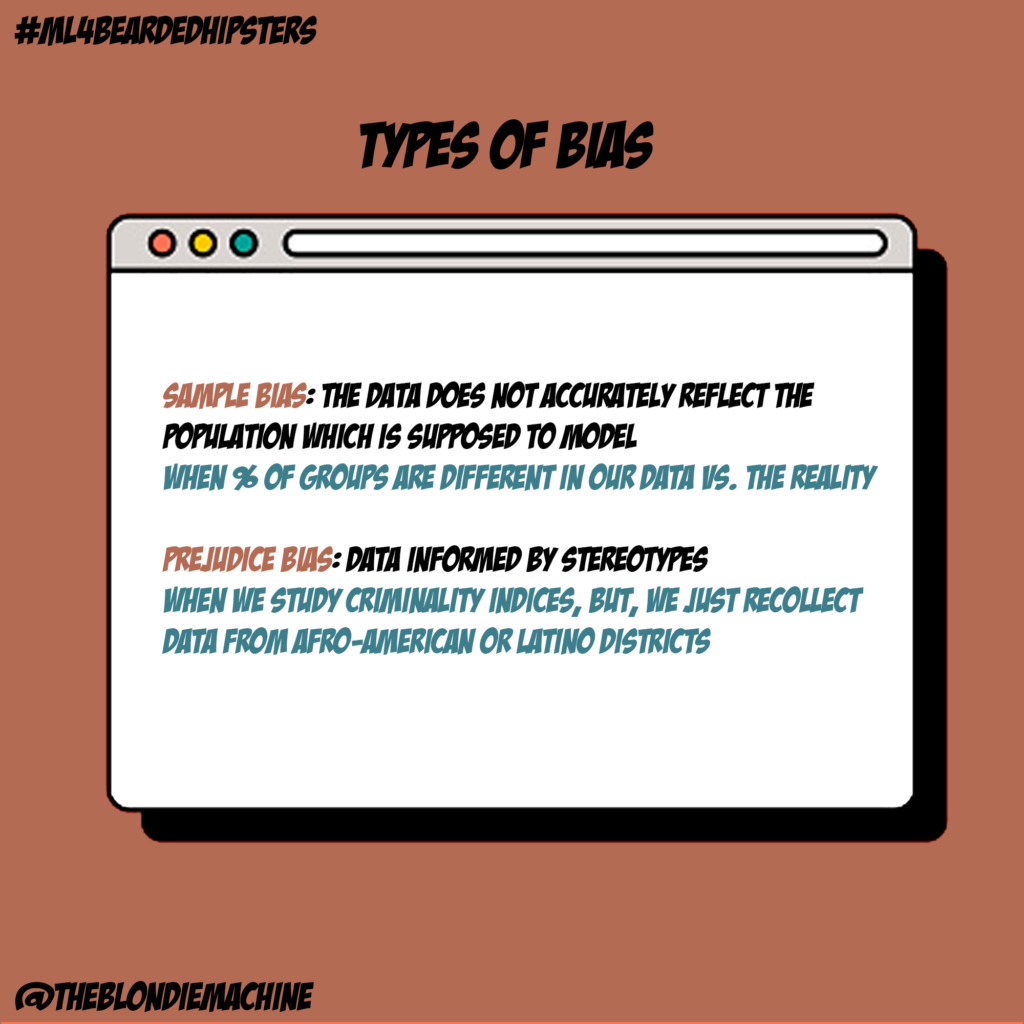
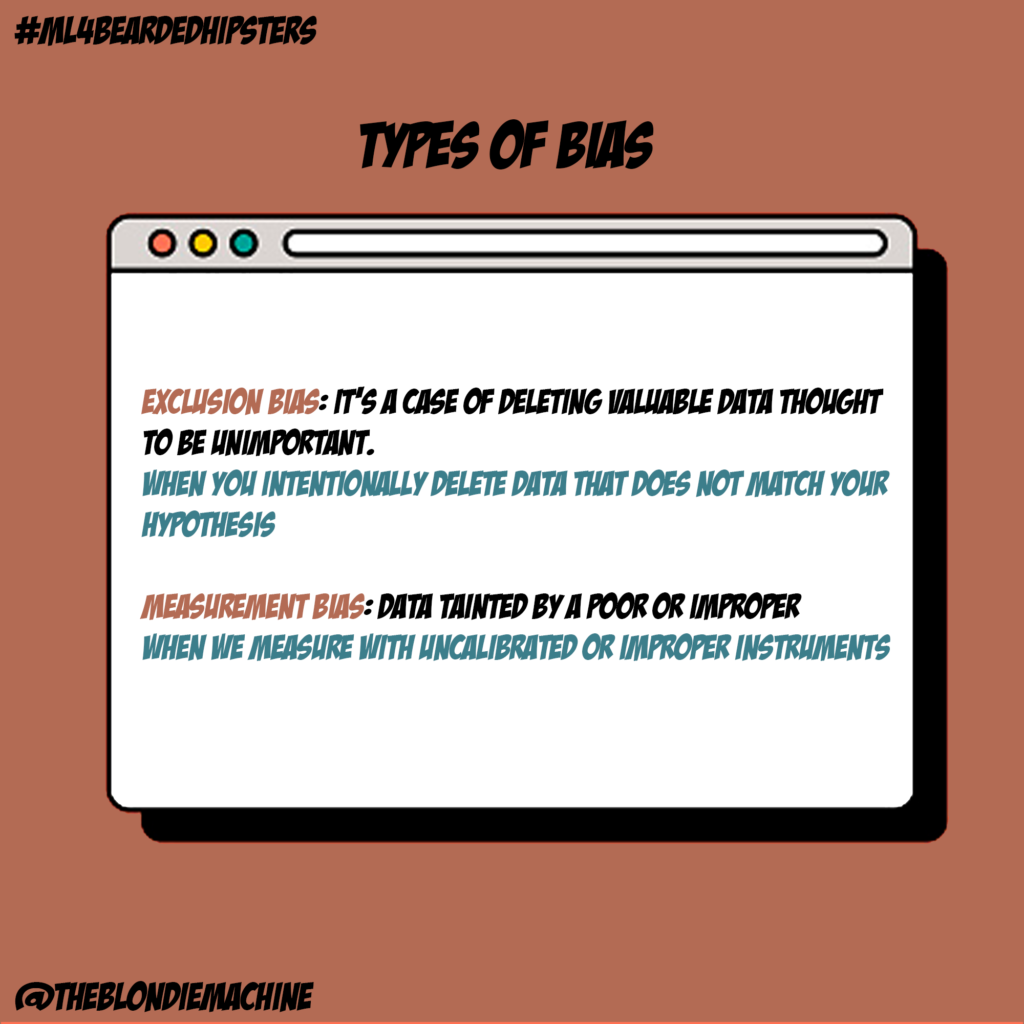
Sample Bias: the data does not accurately reflect the population which is supposed to model
When % of groups are different in our data vs. the reality
Prejudice Bias: Data informed by stereotypes
When we study criminality indices, but, we just recollect data from Afro-American or Latino districts
Exclusion Bias: it’s a case of deleting valuable data thought to be unimportant.
When you intentionally delete data that does not match your hypothesis
Measurement Bias: Data tainted by a poor or improper
When we measure with uncalibrated or improper instruments
How to avoid bias?
- Identify accurate representative data as training set
- Document and share how data is selected and cleansed
- Monitor performance using real data
- Perform subpopulation analysis
- Use tools to analyze the bias (like IBM AI Fairness 360 and Google’s What-If tool)
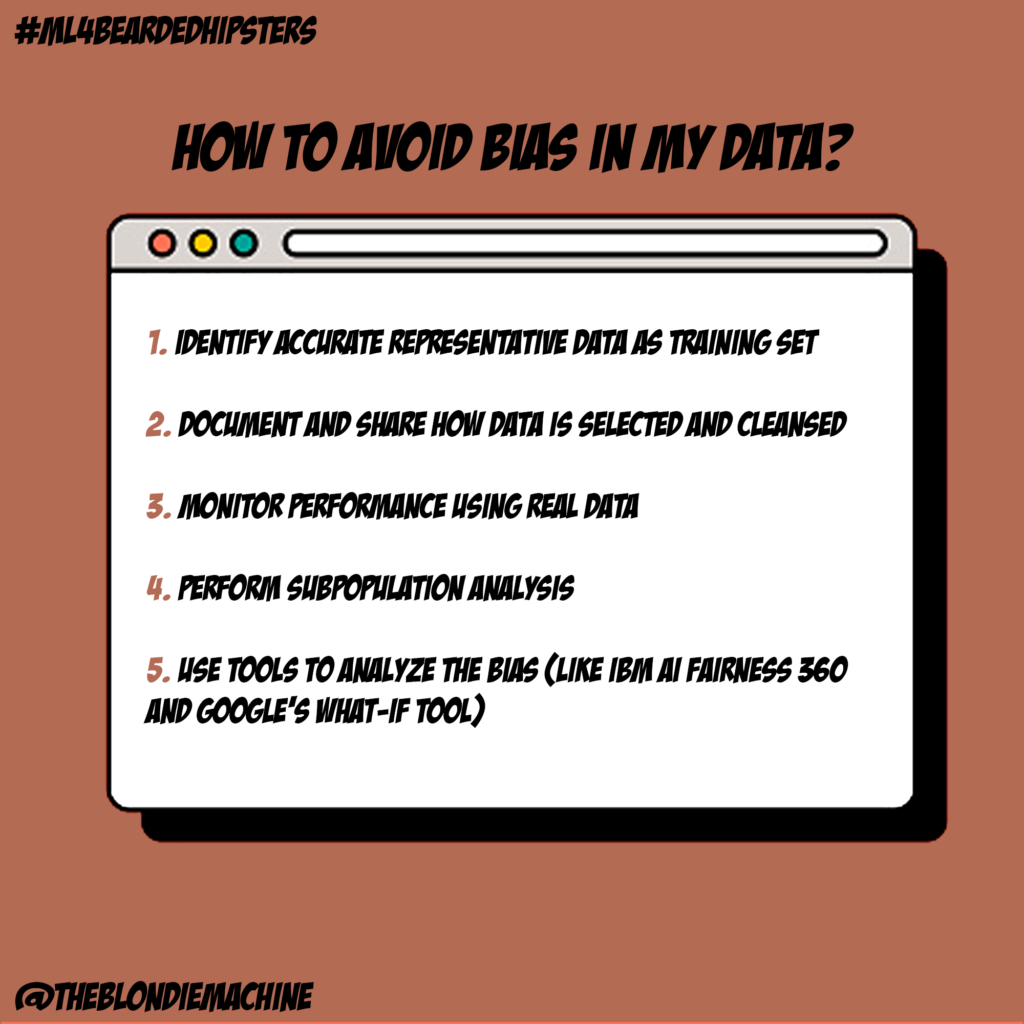
And last of all…
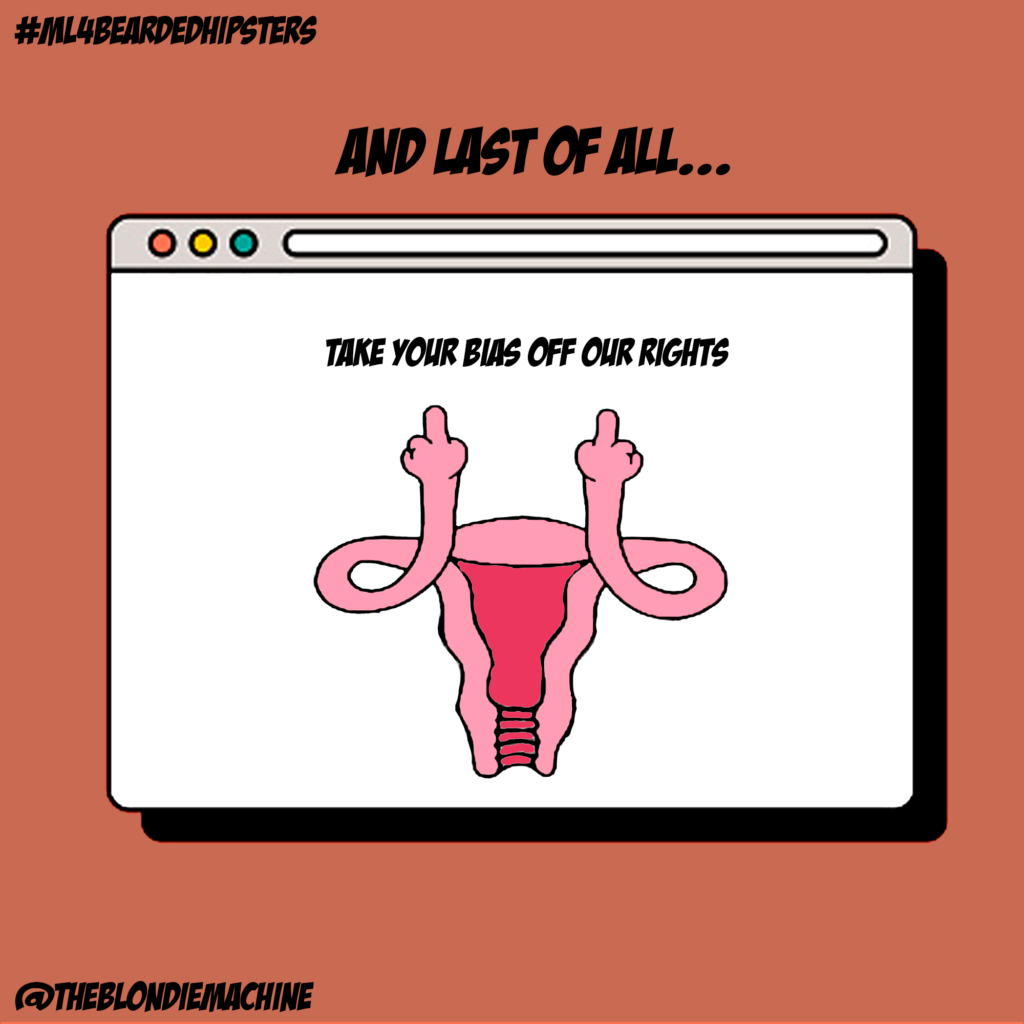
And last but not less important, don’t let your bias affects anyone’s rights!
If you like our work, consider support us as a Patreon
If you are a company related to Academia, Computer Vision, or Machine Learning and want to sponsor one of our comics, read this page about TBM sponsorship.